Predicting the Length of Stay among Healthcare Workers in Underserved Communities: A Quantitative Retrospective Cohort Study
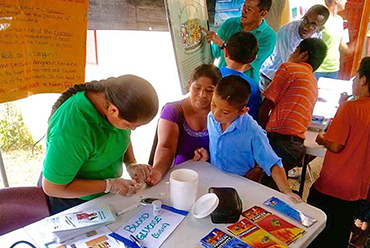
Abstract:
Background: While prior studies have identified a number
of demographic factors related to general health practitioners’ decision to stay
in public health practice, recruitment agencies have no validated methods to predict
how long these health workers will commit to their placement. We aim to use machine
learning methods to predict health professional’s length of practice in the rural
public healthcare sector.
Methods: Recruitment and retention data from Africa
Health Placements (n=13 698 with 1 838 completers) was used to development machine
learning models to predict health workers’ length of practice. A cross-validation
technique was used to validate the models, to evaluate which model performs better,
based on their respective aggregated error rate of prediction. Length of stay was
categorised into 4 groups (less than 1 year, less than 2 years, less than 3 years,
and more than 3 years). Three machine learning models were trained and used 10-fold
cross validation techniques to attain evaluative statistics.
Results: The three models attain almost identical
results, with negligible difference in accuracy. The ‘best’-performing model (Multinomial
logistic classifier) achieved a 47.34% [SD 1.63] while the decision tree model achieved
an almost comparable 45.82% [SD 1.69]. The three models achieved the average AUC
of approximately 0.66 suggesting sufficient predictive signal at the four categorical
variables selected.
Conclusions: Machine learning models give us an effective
tool to predict the recruited health workers’ length of practice. These models can
be adapted beyond the scope of demographic information such as information about
placement location and income. This modelling will also, allow strategic planning
and optimization of public health care recruitment.
Key message
Human resource planning in healthcare can employ machine learning to effectively predict length of stay of recruited health workers who are stationed rural areas.
References:
[1]. Alhassan RK, Spieker N, van Ostenberg P, Ogink A,
Nketiah-Amponsah E, de Wit TFR. Association between health worker motivation
and healthcare quality efforts in Ghana. Hum Resour Health. 2013; 11(1):37.
doi: 10.1186/1478-4491-11-37.
[2]. Agyepong IA, Anafi P, Asiamah E, et al. Health worker
(internal customer) satisfaction and motivation in the public sector in Ghana.
Hum Resour Heal. 2012; 11(247). doi: 10.1186/1472-698X-12-25.
[3]. Buchan J, Couper ID, Tangcharoensathien V, et al. Early
implementation of WHO recommendations for the retention of health workers in
remote and rural areas. Bull World Health Organ. 2013; 91(11):834-840.
doi:10.2471/BLT.13.119008. NDoH. National Health Insurance; 2017.
[4]. Bangdiwala IS, Fonn S, Okoye O, Tollman S. Workforce
Resources for Health in Developing Countries. Public Heal Rev. 2010;
32(1):296-318.
[5]. Cometto G, Tulenko K, Muula AS, Krech R. Health
Workforce Brain Drain: From Denouncing the Challenge to Solving the Problem.
PLoS Med. 2013; 10(9). doi:10.1371/journal.pmed.1001514.
[6]. Dovlo D. The Brain Drain and Retention of Health
Professionals in Africa. A case study Prep a Reg Train Conf Improv Tert Educ
sub-Saharan Africa Things that Work. 2003:23–25.
[7]. Delobelle P, Rawlinson JL, Ntuli S, Malatsi I, Decock
R, Depoorter AM. Job satisfaction and turnover intent of primary healthcare
nurses in rural South Africa: A questionnaire survey. J Adv Nurs.
2011;67(2):371-383. doi:10.1111/j.1365-2648.2010.05496.x.
[8]. George G, Gow J, Bachoo S. Understanding the factors
influencing health-worker employment decisions in South Africa. Hum Resour
Health. 2013; 11(1):15. doi: 10.1186/1478-4491-11-15.
[9]. Hand DJ. A Simple Generalisation of the Area under the
ROC Curve for Multiple Class Classification Problems. Mach Learn. 2001:171-186.
[10]. Habte, D., Dussault, G., Dovlo D. Challenges
confronting the health workforce in Sub-Saharan Africa. World Hosp Heal Serv.
2004; 40(2):23-26. https://www.researchgate.net/profile/Gilles_Dussault/publication/8373533_Challenges_confronting_the_health_workforce_in_sub-Saharan_Africa/links/0fcfd510c3af1833e7000000.pdf#page=22.
[11]. Hatcher AM, Onah M, Kornik S, Peacocke J, Reid S.
Placement, support, and retention of health professionals: national,
cross-sectional findings from medical and dental community service officers in
South Africa. Hum Resour Health. 2014; 12(1):12:14. Doi: 10.1186/1478-4491-12-14.
[12]. Kok MC, Dieleman M, Taegtmeyer M, et al. Which
intervention design factors influence performance of community health workers
in low- and middle-income countries? A systematic review. Health Policy Plan.
2014; 30(9):1207-1227. doi:10.1093/heapol/czu126.
[13]. Landis JR, Koch GG. An Application of Hierarchical
Kappa-type Statistics in the Assessment of Majority Agreement among Multiple
Observers. Biometrics. 1977; 33(2):363. doi: 10.2307/2529786.
[14]. Labonté R, Sanders D, Mathole T, et al. Health worker
migration from South Africa: causes, consequences and policy responses. Hum
Resour Health. 2015; 13(1):92. doi: 10.1186/s12960-015-0093-4.
[15]. Rosenthal EL, Brownstein JN, Rush CH, et al. Community
health workers: part of the solution. Health Aff (Millwood). 2010;
29(7):1338-1342. doi:10.1377/hlthaff.2010.0081.
[16]. Steinmetz S, Vries DH de, Tijdens KG. Should I stay or
should I go? The impact of working time and wages on retention in the health
workforce. Hum Resour Health. 2014; 12(1):23. doi: 10.1186/1478-4491-12-23.
[17]. Sieleunou I. Health worker migration and universal
health care in Sub-Saharan Africa. Pan Afr Med J. 2011; 10: 55. http://www.ncbi.nlm.nih.gov/pubmed/22384301%5Cn
http://www.pubmedcentral.nih.gov/articlerender.fcgi?artid=PMC3290885.
[18]. Viscomi M, Larkins S, Sen Gupta T. Recruitment and
retention of general practitioners in rural Canada and Australia: a review of
the literature. Can J Rural Med. 2013; 18(1):13-24.