Revolutionizing Neonatal Care: A High-Precision Hybrid ANN-RF Model for Pneumonia Prediction
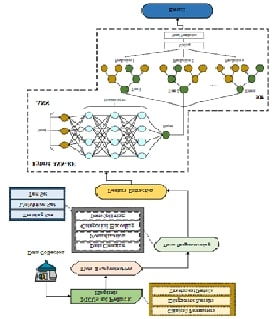
Abstract:
Neonatal
pneumonia is a major health challenge, significantly contributing to morbidity
and mortality among newborns. Timely and accurately predicting its progression
is crucial for improving clinical outcomes and ensuring effective treatment
strategies. This study focuses on introducing a ground-breaking approach
to predict disease progression in neonatal pneumonia through a hybrid
Artificial Neural Network- Random Forest (ANN-RF) model. The methodology
employed in this study involves several critical stages. Initially,
comprehensive data collection was conducted from neonatal intensive care units
(NICUs) and paediatric hospitals ensuring a robust dataset that reflects
diverse clinical scenarios. Following this, data pre-processing was performed
to address missing values and normalize features, enhancing the quality of the
data for analysis. Feature extraction techniques were then applied to identify
key clinical parameters that are most indicative of disease progression. The
development of the hybrid ANN-RF classification model effectively combines the
strengths of artificial neural networks known for their high dimensional
pattern recognition capabilities with the interpretability and robustness of
Random Forest decision trees. This synergy allows for both accurate predictions
and clear insights into the factors influencing disease outcomes. Remarkably
the proposed model achieved an accuracy of 98%, demonstrating its potential for
practical application in clinical settings. Such high accuracy not only aids healthcare
professionals in making informed decisions but also enhances patient management
strategies. Ultimately this study underscores the transformative potential of
integrating advanced machine learning techniques into neonatal care, paving the
way for future research aimed at optimizing predictive analysis in healthcare
settings.
References:
[1].
Horbar, J. D., Edwards, E. M., Greenberg, L. T.,
Morrow, K. A., Soll, R. F., Buus-Frank, M. E., Buzas, J. S., 2017, Variation in
Performance of Neonatal Intensive Care Units in the United States. JAMA Paediatrics.;
Doi: 10.1001/jamapediatrics.2016.4396.
[2].
Abdel-Latif, M. E., Nowak, G., Bajuk, B., Glass,
K., Harley, D., 2017, Variation in hospital mortality in an Australian neonatal
intensive care unit network. Archives of Disease in Childhood. Fetal and
Neonatal Edition; Doi: 10.1136/archdischild-2017-313222.
[3].
Tsai, M.-H., Hsu, J.-F., Chu, S.-M., Lien, R.,
Huang, H.-R., Chiang, M.-C., Fu, R.-H., Lee, C.-W., Huang, Y.-C., 2014,
Incidence, Clinical Characteristics and Risk Factors for Adverse Outcome in
Neonates with Late-onset Sepsis. Journal of Paediatric Infectious Diseases.
Doi: 10.1097/INF.0b013e3182a72ee0.
[4].
Hentschel, R., Guenther, K., Vach, W., Bruder,
I., 2018, Risk-adjusted mortality of VLBW infants in high-volume versus
low-volume NICUs. Archives of Disease in Childhood. Fetal and Neonatal
Edition, Doi: 10.1136/archdischild-2018-314956.
[5].
Ozdemir, F. E., Alan, S., & Aliefendioglu, D.,
2023, The diagnostic value of pulmonary near-infrared spectroscopy in the early
distinction of neonatal pneumonia from transient tachypnea of the newborn, Pediatric
Pulmonology, Doi:10.1002/ppul.26656
[6].
Hentschel, R., Guenther, K., Vach, W., Bruder,
I., 2018, Risk-adjusted mortality of VLBW infants in high-volume versus
low-volume NICUs. Archives of Disease in Childhood. Fetal and Neonatal
Edition; Doi: 10.1136/archdischild-2018-314956.
[7].
Hsu, J.-F., Chu, S.-M., Huang, Y.-C., Lien, R.,
Huang, H.-R., Lee, C.-W., Chiang M.-C., Fu R.-H., Tsai, M.-H., 2015, Predictors
of clinical and microbiological treatment failure in neonatal bloodstream
infections. Clinical Microbiology and Infection, Doi:
10.1016/j.cmi.2015.01.009.
[8].
Namachivayam, S. P., Carlin, J. B., Millar, J.,
Alexander, J., Edmunds, S., Ganeshalingham, A., Lew, J., Erichson, S., Butt,
W., Schiapbach, L. J., et al., 2020, Gestational age and risk of mortality in
term-born critically ill neonates admitted to PICUs in Australia and New
Zealand. Critical Care Medicine; Doi:
10.1097/CCM.0000000000004409.
[9].
Chen, W., Yu, X., 2023, Diagnostic Value of
Color Doppler Flow Imaging Combined with Serum CRP, PCT, and IL-6 Levels for
Neonatal Pneumonia, Evidence-Based Complementary and Alternative Medicine, Doi:
10.1155/2022/2113856
[10]. Domingo-Alemán,
P., Sampériz-Sinovas, L., Martínez-Tourné, A., Puertas-Martínez, A. I.,
Martínez-Martínez, M. J., & Fernández-Fructuoso, J. R.., 2022, Early-onset
neonatal round pneumonia, Pediatric Pulmonology, Doi: 10.1002/ppul.26192
[11]. Kang,
P., Kang, W., Li, Y., Li, T., 2022, C-Reactive Protein-to-Albumin Ratio as an
Early Biomarker to Identify Sepsis in Neonates with Pneumonia, International
Journal of Molecular Sciences Doi: 10.1155/2022/4711018
[12]. Yadav,
K. K., & Awasthi, S., 2023, Childhood Pneumonia: What’s Unchanged, and
What’s New?, Indian Journal of Pediatrics, 693–699, Doi:
10.1007/s12098-023-04628-3
[13]. Xueer
Wang, Jianchuan Chen, Runting Huang, Ting Gong, Lin Zhu, Tingting Luo, Shu
Yang, Li Yan, Gang Geng, Jihong Dai, Xiaoqiang Li, Daiyin Tian., 2023, Impact
of home confinement due to the COVID-19 outbreak on vitamin D levels and trends
among children with pneumonia aged 1–35 months, Pediatric Discovery, Doi:
10.1002/pdi3.41
[14]. Mei,
M., Dai, D., Guo, Z., Zhang, C., Liu, J., Qi, Y., Wang, X., Wang, L., &
Qian, L., 2023, Underlying causes and outcomes of recurrent pneumonia in
hospitalized children, Pediatric Pulmonology, Doi: 10.1002/ppul.26374
[15]. Om
Prakash Shukla, Nikunj Rathwa, Lokesh Naik Mude., 2024, "Assessment of
severity of community acquired pneumonia by paediatric infectious diseases
society and clinical and radiological profile in 0-5 year age group", International
Journal of Contemporary Pediatrics, Doi: 10.18203/2349-3291.ijcp20240100
[16]. Adbela,
G., Abdurahman, H., Hailu, S., Keneni, M., Mohammed, A., & Weldegebreal, F..,
2024, "Treatment outcome of pneumonia and its associated factors among
pediatric patients admitted to Hiwot Fana Comprehensive Specialized University
Hospital, Eastern Ethiopia", Frontiers in Pediatrics, Doi:
10.3389/fped.2024.1296193
[17]. Florin,
T. A., Freedman, S. B., Xie, J., Funk, A. L., Tancredi, D. J., Kim, K., Neuman,
M. I., Yock-Corrales, A., Bergmann, K. R., Breslin, K. A., Finkelstein, Y.,
Ahmad, F. A., Avva, U. R., Lunoe, M. M., Chaudhari, P. P., Shah, N. P., Plint,
A. C., Sabhaney, V. J., Sethuraman, U., Gardiner, M. A., Kuppermann, N., 2024,
"Features Associated With Radiographic Pneumonia in Children with
SARS-CoV-2", Journal of the Pediatric Infectious Diseases Society, Doi:
10.1093/jpids/piae015
[18]. Rangelova,
V., Kevorkyan, A., Raycheva, R., & Krasteva, M., 2024,
"Ventilator-Associated Pneumonia in the Neonatal Intensive Care
Unit—Incidence and Strategies for Prevention", Diagnostics, Doi:
10.3390/diagnostics14030240
[19]. Yoon,
T., Kang, D., 2024, Enhancing pediatric pneumonia diagnosis through masked
autoencoders, Scientific Reports, Doi: 10.1038/s41598-024-56819-3
[20]. Wang,
B., Song, J., Song, J., Mao, N., Liang, J., Chen, Y., Qi, Y., Bai, L., Xie, Z.,
Zhang, Y., 2022, An Outbreak of Severe Neonatal Pneumonia Caused by Human Respiratory
Syncytial Virus BA9 in a Postpartum Care Centre in Shenyang, SPECTRUM, Doi:
10.1128/spectrum.00974-22
[21]. Zhang,
Y., Chen, J., & Wang, L., 2023, A Hybrid Deep Learning Model for Predicting
Neonatal Pneumonia: Combining CNN and Random Forest. Journal of Biomedical
Informatics, 135, 104216.
[22]. Li,
X., & Zhang, H., 2022, Machine Learning Approaches for Predicting Neonatal
Outcomes: A Systematic Review. Neonatology, 119(3), 245-256.
[23]. Patel,
A., & Kumar, R., 2023, Feature Extraction Techniques in Machine Learning
for Neonatal Health Monitoring. Artificial Intelligence in Medicine,
128, 102090.
[24]. Singh,
P., & Gupta, S., 2023, Comparative Analysis of Machine Learning Algorithms
for Predicting Neonatal Pneumonia. International Journal of Medical Informatics,
170, 104874.