Application of Artificial Intelligence (AI) as the Assisting Strategy in the Cardiology Department
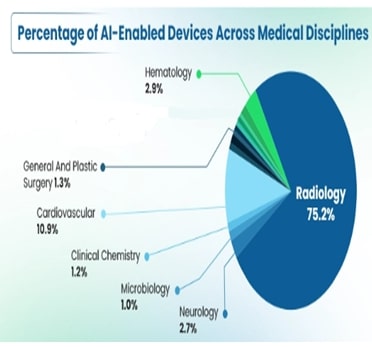
Abstract:
This manuscript explores the application of artificial intelligence (AI)
in the cardiology department, highlighting its transformative impact on
diagnostics, treatment, and patient management. AI encompasses various methods,
including machine learning and deep learning, which enable the analysis of
extensive data sets for improved decision-making in clinical practice. The
paper discusses how the development of AI technologies that enhance the
identification and prediction of cardiovascular diseases, through innovative
analytical solutions that offer superior accuracy and speed compared to
traditional methods. A significant focus is placed on AI’s capability to
swiftly interpret complex ECG patterns, facilitating early diagnosis of
life-threatening arrhythmias. Furthermore, the manuscript emphasizes the
importance of personalized medicine, wherein AI-driven insights contribute to
tailored care plans for individual patients. The strategic integration of AI
within cardiology not only enhances the quality of care but also streamlines
clinical workflows. By addressing ethical considerations and potential biases,
this paper aims to provide a comprehensive overview of the current state and
future potential of AI in cardiology, ultimately advocating for its wider
implementation to advance patient outcomes in cardiovascular health.
References:
[1].
Harmon,
D. M., Mangold, K., Baez Suarez, A., Scott, C. G., Murphree, D. H., Malik, A.,
Attia, Z. I., Lopez-Jimenez, F., Friedman, P. A., Dispenzieri, A., &
Grogan, M. 2023, Post development Performance and Validation of the Artificial
Intelligence-Enhanced Electrocardiogram for Detection of Cardiac Amyloidosis. JACC:
Advances, 2(8), 100612. https://doi.org/10.1016/j.jacadv.2023.100612
[2].
Williams,
R., Anderson, S., Cresswell, K., Kannelønning, M. S., Mozaffar, H., & Yang,
X. 2024, Domesticating AI in medical diagnosis. Technology in Society,
76, 102469. https://doi.org/10.1016/j.techsoc.2024.102469
[3].
Huang,
K.-C., Huang, C.-S., Su, M.-Y., Hung, C.-L., Ethan Tu, Y.-C., Lin, L.-C., &
Hwang, J.-J. 2021, Artificial Intelligence Aids Cardiac Image Quality
Assessment for Improving Precision in Strain Measurements. JACC:
Cardiovascular Imaging, 14(2), 335–345. https://doi.org/10.1016/j.jcmg.2020.08.034
[4].
Adedinsewo,
D. A., Pollak, A. W., Phillips, S. D., Smith, T. L., Svatikova, A., Hayes, S.
N., Mulvagh, S. L., Norris, C., Roger, V. L., Noseworthy, P. A., Yao, X., &
Carter, R. E. 2022, Cardiovascular Disease Screening in Women: Leveraging
Artificial Intelligence and Digital Tools. Circulation Research, 130(4),
673–690. https://doi.org/10.1161/CIRCRESAHA.121.319876
[5].
Alharbi,
Y. 2024, Artificial intelligence in cardiology: Present state and prospective
directions. Journal of Radiation Research and Applied Sciences, 17(3),
101012. https://doi.org/10.1016/j.jrras.2024.101012
[6].
Attia,
Z. I., Harmon, D. M., Behr, E. R., & Friedman, P. A. 2021, Application of
artificial intelligence to the electrocardiogram. European Heart Journal,
42(46), 4717–4730. https://doi.org/10.1093/eurheartj/ehab649
[7].
Balloch,
J., Sridharan, S., Oldham, G., Wray, J., Gough, P., Robinson, R., Sebire, N.
J., Khalil, S., Asgari, E., Tan, C., Taylor, A., & Pimenta, D. 2024, Use of
an ambient artificial intelligence tool to improve the quality of clinical
documentation. Future Healthcare Journal, 11(3), 100157. https://doi.org/10.1016/j.fhj.2024.100157
[8].
Bahado-Singh,
R., Friedman, P., Talbot, C., Aydas, B., Southekal, S., Mishra, N. K., Guda,
C., Yilmaz, A., Radhakrishna, U., & Vishweswaraiah, S. 2023, Cell-free DNA
in maternal blood and artificial intelligence: Accurate prenatal detection of
fetal congenital heart defects. American Journal of Obstetrics and
Gynecology, 228(1), 76.e1-76.e10. https://doi.org/10.1016/j.ajog.2022.07.062
[9].
Brendel,
J. M., Walterspiel, J., Hagen, F., Kübler, J., Paul, J.-F., Nikolaou, K.,
Gawaz, M., Greulich, S., Krumm, P., & Winkelmann, M. 2024, Coronary artery
disease evaluation during transcatheter aortic valve replacement work-up using
photon-counting CT and artificial intelligence. Diagnostic and
Interventional Imaging, 105(7–8), 273–280. https://doi.org/10.1016/j.diii.2024.01.010
[10]. Chen, E., Jiang, J., Su, R., Gao, M., Zhu, S., Zhou, J.,
& Huo, Y. 2020, A new smart wristband equipped with an artificial
intelligence algorithm to detect atrial fibrillation. Heart Rhythm,
17(5), 847–853. https://doi.org/10.1016/j.hrthm.2020.01.034
[11]. De Koning, E., Van der Haas, Y., Saguna, S., Stoop, E.,
Bosch, J., Beeres, S., Schalij, M., & Boogers, M. 2023, AI Algorithm to
Predict Acute Coronary Syndrome in Prehospital Cardiac Care: Retrospective
Cohort Study. JMIR Cardio, 7, e51375. https://doi.org/10.2196/51375
[12]. Dey, D., Slomka, P. J., Leeson, P., Comaniciu, D., Shrestha,
S., Sengupta, P. P., & Marwick, T. H. 2019, Artificial Intelligence in
Cardiovascular Imaging. Journal of the American College of Cardiology,
73(11), 1317–1335. https://doi.org/10.1016/j.jacc.2018.12.054
[13]. Echefu, G., Shah, R., Sanchez, Z., Rickards, J., &
Brown, S.-A. 2024, Artificial intelligence: Applications in cardio-oncology and
potential impact on racial disparities. American Heart Journal Plus:
Cardiology Research and Practice, 48, 100479. https://doi.org/10.1016/j.ahjo.2024.100479
[14]. Firima, E., Gonzalez, L., Manthabiseng, M., Bane, M., Lukau,
B., Leigh, B., Kaufmann, B. A., Weisser, M., Amstutz, A., Tromp, J., Labhardt,
N. D., & Burkard, T. 2024, Implementing focused echocardiography and
AI-supported analysis in a population-based survey in Lesotho: Implications for
community-based cardiovascular disease care models. Hypertension Research,
47(3), 708–713. https://doi.org/10.1038/s41440-023-01559-6
[15]. Gearhart, A., Dwork, N., & Jone, P.-N. 2022, Artificial
intelligence in echocardiography to diagnose congenital heart disease and fetal
echocardiography. Intelligence-Based Medicine, 6, 100082. https://doi.org/10.1016/j.ibmed.2022.100082
[16]. Haleem, A., Javaid, M., Singh, R. P., & Suman, R. 2021,
Applications of Artificial Intelligence (AI) for cardiology during the COVID-19
pandemic. Sustainable Operations and Computers, 2, 71–78. https://doi.org/10.1016/j.susoc.2021.04.003
[17]. In Kim, Y., Roh, J.-H., Kweon, J., Kwon, H., Chae, J., Park,
K., Lee, J.-H., Jeong, J.-O., Kang, D.-Y., Lee, P. H., Ahn, J.-M., Kang, S.-J.,
Park, D.-W., Lee, S.-W., Lee, C. W. W., Park, S.-J., & Kim, Y.-H. 2024,
Artificial intelligence-based quantitative coronary angiography of major
vessels using deep-learning. International Journal of Cardiology, 405,
131945. https://doi.org/10.1016/j.ijcard.2024.131945
[18]. Johnson, K. W., Torres Soto, J., Glicksberg, B. S., Shameer,
K., Miotto, R., Ali, M., Ashley, E., & Dudley, J. T. 2018, Artificial
Intelligence in Cardiology. Journal of the American College of Cardiology,
71(23), 2668–2679. https://doi.org/10.1016/j.jacc.2018.03.521
[19]. Wang, Y.-R., Yang, K., Wen, Y., Wang,
P., Hu, Y., Lai, Y., Wang, Y., Zhao, K., Tang, S., Zhang, A., Zhan, H., Lu, M.,
Chen, X., Yang, S., Dong, Z., Wang, Y., Liu, H., Zhao, L., Huang, L., … Zhao, S. 2024, Screening and diagnosis of cardiovascular
disease using artificial intelligence-enabled cardiac magnetic resonance
imaging. Nature Medicine, 30(5), 1471–1480. https://doi.org/10.1038/s41591-024-02971-2
[20]. Jones, L. K., Gidding, S. S., Santos, R. D., Libby, P.,
Watts, G. F., & Sarkies, M. N. 2024, Application of implementation science
for improving the utilization of an international clinical practice guidance on
familial hypercholesterolemia. Journal of Clinical Lipidology, 18(4),
e525–e536. https://doi.org/10.1016/j.jacl.2024.03.010
[21]. Kassem, K., Sperti, M., Cavallo, A., Vergani, A. M.,
Fassino, D., Moz, M., Liscio, A., Banali, R., Dahlweid, M., Benetti, L., Bruno,
F., Gallone, G., De Filippo, O., Iannaccone, M., D’Ascenzo, F., De Ferrari, G.
M., Morbiducci, U., Della Valle, E., & Deriu, M. A. 2024, An innovative
artificial intelligence-based method to compress complex models into
explainable, model-agnostic and reduced decision support systems with
application to healthcare (NEAR). Artificial Intelligence in Medicine,
151, 102841. https://doi.org/10.1016/j.artmed.2024.102841
[22]. Liu, C.-M., Shih, E. S. C., Chen, J.-Y., Huang, C.-H., Wu,
I.-C., Chen, P.-F., Higa, S., Yagi, N., Hu, Y.-F., Hwang, M.-J., & Chen,
S.-A. 2022, Artificial Intelligence-Enabled Electrocardiogram Improves the
Diagnosis and Prediction of Mortality in Patients With Pulmonary Hypertension. JACC:
Asia, 2(3), 258–270. https://doi.org/10.1016/j.jacasi.2022.02.008
[23]. Lin, F., Zhang, P., Chen, Y., Liu, Y., Li, D., Tan, L.,
Wang, Y., Wang, D. W., Yang, X., Ma, F., & Li, Q. 2024,
Artificial-intelligence-based risk prediction and mechanism discovery for
atrial fibrillation using heartbeat-to-beat intervals. Med, 5(5),
414-431.e5. https://doi.org/10.1016/j.medj.2024.02.006
[24]. Kirchner, J., Gerçek, M., Gesch, J., Omran, H., Friedrichs,
K., Rudolph, F., Ivannikova, M., Rossnagel, T., Piran, M., Pfister, R., Blanke,
P., Rudolph, V., & Rudolph, T. K. 2024, Artificial intelligence-analyzed
computed tomography in patients undergoing transcatheter tricuspid valve
repair. International Journal of Cardiology, 411, 132233. https://doi.org/10.1016/j.ijcard.2024.132233
[25]. Krishna, H., Desai, K., Slostad, B., Bhayani, S., Arnold, J.
H., Ouwerkerk, W., Hummel, Y., Lam, C. S. P., Ezekowitz, J., Frost, M., Jiang,
Z., Equilbec, C., Twing, A., Pellikka, P. A., Frazin, L., & Kansal, M. 2023,
Fully Automated Artificial Intelligence Assessment of Aortic Stenosis by
Echocardiography. Journal of the American Society of Echocardiography,
36(7), 769–777. https://doi.org/10.1016/j.echo.2023.03.008
[26]. Krittanawong, C., Johnson, K. W., Choi, E., Kaplin, S.,
Venner, E., Murugan, M., Wang, Z., Glicksberg, B. S., Amos, C. I., Schatz, M.
C., & Tang, W. H. W. 2022, Artificial Intelligence and Cardiovascular Genetics.
Life, 12(2), 279. https://doi.org/10.3390/life12020279
[27]. Krittanawong, C., Zhang, H., Wang, Z., Aydar, M., &
Kitai, T. 2017, Artificial Intelligence in Precision Cardiovascular Medicine. Journal
of the American College of Cardiology, 69(21), 2657–2664. https://doi.org/10.1016/j.jacc.2017.03.571
[28]. Ledziński, Ł., & Grześk, G. 2023, Artificial
Intelligence Technologies in Cardiology. Journal of Cardiovascular
Development and Disease, 10(5), 202. https://doi.org/10.3390/jcdd10050202
[29]. Vrudhula, A., Stern, L., Cheng, P. C., Ricchiuto, P.,
Daluwatte, C., Witteles, R., Patel, J., & Ouyang, D. 2024, Impact of Case
and Control Selection on Training Artificial Intelligence Screening of Cardiac
Amyloidosis. JACC: Advances, 3(9), 100998. https://doi.org/10.1016/j.jacadv.2024.100998
[30]. Wang, H., Zu, Q., Lu, M., Chen, R., Yang, Z., Gao, Y., &
Ding, J. 2022, Application of Medical Knowledge Graphs in Cardiology and
Cardiovascular Medicine: A Brief Literature Review. Advances in Therapy,
39(9), 4052–4060. https://doi.org/10.1007/s12325-022-02254-7
[31]. Li, F., Bi, Z., Xu, H., Shi, Y., Duan, N., & Li, Z. 2023,
Design and implementation of a smart Internet of Things chest pain center based
on deep learning. Mathematical Biosciences and Engineering, 20(10),
18987–19011. https://doi.org/10.3934/mbe.2023840
[32]. Matthiesen, S., Diederichsen, S. Z., Hansen, M. K. H.,
Villumsen, C., Lassen, M. C. H., Jacobsen, P. K., Risum, N., Winkel, B. G.,
Philbert, B. T., Svendsen, J. H., & Andersen, T. O. 2021, Clinician Reimplementation
Perspectives of a Decision-Support Tool for the Prediction of Cardiac
Arrhythmia Based on Machine Learning: Near-Live Feasibility and Qualitative
Study. JMIR Human Factors, 8(4), e26964. https://doi.org/10.2196/26964
[33]. Lin, C., Chen, C.-C., Chau, T., Lin, C.-S., Tsai, S.-H.,
Lee, D.-J., Lee, C.-C., Shang, H.-S., & Lin, S.-H. 2022, Artificial
intelligence-enabled electrocardiography identifies severe dyscalcemias and has
prognostic value. Clinica Chimica Acta, 536, 126–134. https://doi.org/10.1016/j.cca.2022.09.021
[34]. Moualla, S. K., McCarthy, P. M., Thomas, J. D., Dobbles, M.,
Petrescu, O. M., Loper, T., Barnhart, G. R., & Brennan, J. M. 2022, The
artificial intelligence-enabled predictive model of progression from moderate
to severe aortic stenosis. Intelligence-Based Medicine, 6, 100062. https://doi.org/10.1016/j.ibmed.2022.100062
[35]. Van Tongeren, O. L. R. M., Vanmaele, A., Rastogi, V., Hoeks,
S. E., Verhagen, H. J. M., & De Bruin, J. L. 2024, Volume Measurements for
Surveillance after Endovascular Aneurysm Repair using Artificial Intelligence. European
Journal of Vascular and Endovascular Surgery, S1078588424007883. https://doi.org/10.1016/j.ejvs.2024.08.045
[36]. Vandenberk, B., Chew, D. S., Prasana, D., Gupta, S., &
Exner, D. V. 2023, Successes and challenges of artificial intelligence in
cardiology. Frontiers in Digital Health, 5, 1201392. https://doi.org/10.3389/fdgth.2023.1201392
[37]. Oikonomou, E. K., & Khera, R. 2024, Designing medical
artificial intelligence systems for global use: Focus on interoperability,
scalability, and accessibility. Hellenic Journal of Cardiology,
S1109966624001581. https://doi.org/10.1016/j.hjc.2024.07.003
[38]. Samant, S., Bakhos, J. J., Wu, W., Zhao, S., Kassab, G. S.,
Khan, B., Panagopoulos, A., Makadia, J., Oguz, U. M., Banga, A., Fayaz, M.,
Glass, W., Chiastra, C., Burzotta, F., LaDisa, J. F., Iaizzo, P., Murasato, Y.,
Dubini, G., Migliavacca, F., … Chatzizisis, Y. S. 2023, Artificial
Intelligence, Computational Simulations, and Extended Reality in Cardiovascular
Interventions. JACC: Cardiovascular Interventions, 16(20), 2479–2497 https://doi.org/10.1016/j.jcin.2023.07.022
[39]. Schepart, A., Burton, A., Durkin, L., Fuller, A., Charap,
E., Bhambri, R., & Ahmad, F. S. 2023, Artificial intelligence-enabled tools
in cardiovascular medicine: A survey of current use, perceptions, and
challenges. Cardiovascular Digital Health Journal, 4(3), 101–110. https://doi.org/10.1016/j.cvdhj.2023.04.003
[40]. Toprak, B., Solleder, H., Di Carluccio, E., Greenslade, J.
H., Parsonage, W. A., Schulz, K., Cullen, L., Apple, F. S., Ziegler, A.,
Blankenberg, S., Stephensen, L., Brownlee, E., McCormick, E., Fincher, G.,
Hall, E. J., Hancock, R., Gaikwad, N., Gangathimmaiah, V., Hamilton-Craig, C.,
… Then, M. 2024, Diagnostic accuracy of a machine learning algorithm using
point-of-care high-sensitivity cardiac troponin I for rapid rule-out of
myocardial infarction: A retrospective study. The Lancet Digital Health,
6(10), e729–e738. https://doi.org/10.1016/S2589-7500(24)00191-2
[41]. Otaki, Y., Singh, A., Kavanagh, P., Miller, R. J. H.,
Parekh, T., Tamarappoo, B. K., Sharir, T., Einstein, A. J., Fish, M. B., Ruddy,
T. D., Kaufmann, P. A., Sinusas, A. J., Miller, E. J., Bateman, T. M., Dorbala,
S., Di Carli, M., Cadet, S., Liang, J. X., Dey, D., … Slomka, P. J. 2022,
Clinical Deployment of Explainable Artificial Intelligence of SPECT for
Diagnosis of Coronary Artery Disease. JACC: Cardiovascular Imaging,
15(6), 1091–1102. https://doi.org/10.1016/j.jcmg.2021.04.030
[42]. Soh, C. H., Wright, L., Baumann, A., Seidel, B., Yu, C.,
Nolan, M., Mylius, T., & Marwick, T. H. 2024, Use of artificial
intelligence‐guided echocardiography to detect cardiac dysfunction and heart
valve disease in rural and remote areas: Rationale and design of the AGILE‐echo
trial. American Heart Journal, 277, 11–19. https://doi.org/10.1016/j.ahj.2024.08.004
[43]. Spielvogel, C. P., Haberl, D.,
Mascherbauer, K., Ning, J., Kluge, K., Traub-Weidinger, T., Davies, R. H.,
Pierce, I., Patel, K., Nakuz, T., Göllner, A., Amereller, D., Starace, M.,
Monaci, A., Weber, M., Li, X., Haug, A. R., Calabretta, R., Ma, X., … Nitsche, C. 2024, Diagnosis and prognosis of abnormal
cardiac scintigraphy uptake suggestive of cardiac amyloidosis using artificial
intelligence: A retrospective, international, multicentre, cross-tracer
development and validation study. The Lancet Digital Health, 6(4),
e251–e260. https://doi.org/10.1016/S2589-7500(23)00265-0
[44]. Strom, J. B., Playford, D., Stewart, S., & Strange, G.
2024, An Artificial Intelligence Algorithm for Detection of Severe Aortic
Stenosis. JACC: Advances, 3(9), 101176. https://doi.org/10.1016/j.jacadv.2024.101176
[45]. Palmieri, V., Montisci, A., Vietri, M. T., Colombo, P. C.,
Sala, S., Maiello, C., Coscioni, E., Donatelli, F., & Napoli, C. 2023,
Artificial intelligence, big data, and heart transplantation: Actualities. International
Journal of Medical Informatics, 176, 105110. https://doi.org/10.1016/j.ijmedinf.2023.105110
[46]. Schuuring, M. J., Išgum, I., Cosyns, B., Chamuleau, S. A.
J., & Bouma, B. J. 2021, Routine Echocardiography and Artificial
Intelligence Solutions. Frontiers in Cardiovascular Medicine, 8, 648877.
https://doi.org/10.3389/fcvm.2021.648877
[47]. Shaffer, B. C., Brown, S., Chinapen, S., Mangold, K. E.,
Lahoud, O., Lopez-Jimenez, F., Schaffer, W., Liu, J., Giralt, S., Devlin, S.,
Shah, G., Scordo, M., Papadopoulos, E., Landau, H., Usmani, S., Perales, M.-A.,
Friedman, P. A., Gersh, B. J., Attia, I. Z., … Kosmidou, I. 2024, Artificial intelligence-enabled
interpretation of ECG images to predict hematopoietic cell transplantation
toxicity. Blood Advances, 8(21), 5603–5611. https://doi.org/10.1182/bloodadvances.2024013636
[48]. Rushlow, D. R., Croghan, I. T., Inselman, J. W., Thacher, T.
D., Friedman, P. A., Yao, X., Pellikka, P. A., Lopez-Jimenez, F., Bernard, M.
E., Barry, B. A., Attia, I. Z., Misra, A., Foss, R. M., Molling, P. E., Rosas,
S. L., & Noseworthy, P. A. 2022, Clinician Adoption of an Artificial
Intelligence Algorithm to Detect Left Ventricular Systolic Dysfunction in
Primary Care. Mayo Clinic Proceedings, 97(11), 2076–2085. https://doi.org/10.1016/j.mayocp.2022.04.008
[49]. Salem, M., Gada, H., Ramlawi, B., Sotelo, M., Nona, P.,
Wagner, L., Rogers, C., Brigman, L., & Vora, A. N. 2024, Predictors of
Disease Progression and Adverse Clinical Outcomes in Patients with Moderate
Aortic Stenosis Using an Artificial Intelligence-Based Software Platform. The
American Journal of Cardiology, 223, 92–99. https://doi.org/10.1016/j.amjcard.2024.04.051
[50]. Sveric, K. M., Ulbrich, S., Dindane, Z., Winkler, A., Botan,
R., Mierke, J., Trausch, A., Heidrich, F., & Linke, A. 2024, Improved
assessment of left ventricular ejection fraction using artificial intelligence
in echocardiography: A comparative analysis with cardiac magnetic resonance
imaging. International Journal of Cardiology, 394, 131383. https://doi.org/10.1016/j.ijcard.2023.131383
[51]. Brown, S.-A. 2022, Introduction to “Artificial intelligence
and health informatics in learning health systems in cardio-oncology.” American
Heart Journal Plus: Cardiology Research and Practice, 18, 100156. https://doi.org/10.1016/j.ahjo.2022.100156
[52]. Jasani, M. 2024, AI in Healthcare: A Complete List of the
Latest Statistics. Retrieved from: https://www.excellentwebworld.com/ai-in-healthcare-statistics/
[53]. Page, M. J., McKenzie, J. E., Bossuyt,
P. M., Boutron, I., Hoffmann, T. C., Mulrow, C. D., et al. The PRISMA 2020 statement: An updated guideline for
reporting systematic reviews. BMJ 2021, 372: n71. doi: 10.1136/bmj. n71.