Exploring the Genomic Effects of Pioglitazone on Skeletal Muscle in Polycystic Ovary Syndrome
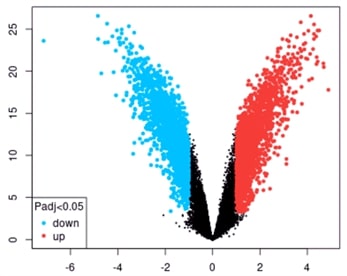
Abstract:
This study examines
the molecular effects of pioglitazone on the skeletal muscle tissue of women
who have polycystic ovary syndrome (PCOS), with an emphasis on the medication's
ability to improve insulin sensitivity and lower inflammation. Differentially
expressed genes (DEGs) that highlight important biological processes and
pathways altered by pioglitazone, such as the cytokine-cytokine receptor
interactions and PI3K-Akt signalling pathway, were found using gene expression
profiling. To predict treatment response and serve as targets for future
pharmaceutical development, the study identified hub genes like ESR1 and KRAS
as key participants in these pathways. These results highlight the complex
function that pioglitazone plays in controlling inflammatory and metabolic
processes, which are essential for the management of PCOS. Although the study
has several merits, such as the thorough molecular analysis, one drawback is
the rather small sample size, which may limit how broadly the results may be
applied. Prospective investigations have to concentrate on verifying our
findings in more extensive cohorts, examining the clinical significance of the
detected biomarkers, and carrying out mechanistic analyses to gain a deeper
comprehension of Pioglitazone's impacts. This work advances our knowledge of
the molecular mechanisms underlying pioglitazone's effects in PCOS, paving the
way for the creation of more individualized and potent treatment plans that
will eventually improve patient outcomes.
References:
[1]. Stener-Victorin, E., Teede, H., Norman, R. J., Legro, R., Goodarzi, M. O., Dokras, A., et al., 2024, Polycystic ovary syndrome. Nat Rev Dis Primers, 10, 27. https://doi.org/10.1038/s41572-024-00511-3
[2]. Al-Janabi, M.A.Y., Bayat, R., Bekmezci, M., et al. 2024, Development of electrochemical immunosensors for early diagnosis of polycystic ovary syndrome (PCOS), and their potential mobile phone application. Top Catal, 67, 688–697. https://doi.org/10.1007/s11244-023-01899-0 10.7759/cureus.40141.
[3]. Shehnaz, S. I., Roy, A., Vijayaraghavan, R., Sivanesan, S., 2023, Luteolin mitigates diabetic dyslipidemia in rats by modulating ACAT-2, PPARα, SREBP-2 proteins, and oxidative stress. Appl Biochem Biotechnol, 195, 4893-4914. doi: 10.1007/s12010-023-04544-4.
[4]. Zhao, H., Zhang, J., Cheng, X., et al., 2023, Insulin resistance in polycystic ovary syndrome across various tissues: an updated review of pathogenesis, evaluation, and treatment. J Ovarian Res, 16, 9. https://doi.org/10.1186/s13048-022-01091-0
[5]. Yki-Jarvinen, H., 2009, Thiazolidinediones and the liver in humans. Curr Opin Lipidol. 20, 477–483. doi: 10.1097/MOL.0b013e3283321d37.
[6]. Brettenthaler, N., De Geyter, C., Huber, P. R., Keller, U., 2004, Effect of the insulin sensitizer pioglitazone on insulin resistance, hyperandrogenism, and ovulatory dysfunction in women with polycystic ovary syndrome. J Clin Endocrinol Metab, 89, 3835–3840. doi: 10.1210/jc.2003-031737.
[7]. Miyazaki, Y., Mahankali, A., Matsuda, M., Glass, L., Mahankali, S., Ferrannini, E., et al., 2001, Improved glycemic control and enhanced insulin sensitivity in type 2 diabetic subjects treated with pioglitazone. Diabetes Care, 24, 710–719. doi: 10.2337/diacare.24.4.710.
[8]. El Fassi, D., Nielsen, C. H., Bonnema, S. J., Hasselbalch, H. C., Hegedüs, L., 2007, B lymphocyte depletion with the monoclonal antibody rituximab in Graves’ disease: a controlled pilot study. J Clin Endocrinol Metab, 92, 1769–1772. doi: 10.1210/jc.2006-2388.
[9]. DeFronzo, R. A., Tripathy, D., Schwenke, D. C., Banerji, M., Bray, G. A., Buchanan, T. A., et al., 2011, Pioglitazone for diabetes prevention in impaired glucose tolerance. N Engl J Med, 364, 1104–1115. doi: 10.1056/NEJMoa1010949.
[10]. Mootha, V. K., Lindgren, C. M., Eriksson, K. F., Subramanian, A., Sihag, S., Lehar, J., et al., 2003, PGC-1alpha-responsive genes involved in oxidative phosphorylation are coordinately downregulated in human diabetes. Nat Genet, 34, 267–273. doi: 10.1038/ng1180.
[11]. Patti, M. E., Butte, A. J., Crunkhorn, S., Cusi, K., Berria, R., Kashyap, S., et al., 2003, Coordinated reduction of genes of oxidative metabolism in humans with insulin resistance and diabetes: Potential role of PGC1 and NRF1. Proc Natl Acad Sci U S A, 100, 8466–8471. doi: 10.1073/pnas.1032913100.
[12]. Pasquali, R., Gambineri, A., 2006, Insulin-sensitizing agents in women with polycystic ovary syndrome. Fertil Steril, 86, S28-9. doi: 10.1016/j.fertnstert.2006.04.012.
[13]. Barrett, T., Wilhite, S. E., Ledoux, P., Evangelista, C., Kim, I. F., Tomashevsky, M., et al., 2013, NCBI GEO: archive for functional genomics data sets--update. Nucleic Acids Res, 41, D991-5. doi: 10.1093/nar/gks1193.
[14]. Skov, V., Glintborg, D., Knudsen, S., Jensen, T., Kruse, T. A., Tan, Q., et al., 2007, Reduced expression of nuclear-encoded genes involved in mitochondrial oxidative metabolism in skeletal muscle of insulin-resistant women with polycystic ovarysyndrome. Diabetes, 56, 2349–2355. doi: 10.2337/db07-0275.
[15]. Reiner, A., Yekutieli, D., Benjamini, Y., 2003, Identifying differentially expressed genes using false discovery rate controlling procedures. Bioinformatics, 19, 368–375. doi: 10.1093/bioinformatics/btf877.
[16]. Benjamini, Y., Hochberg, Y., 1995, Controlling the false discovery rate: A practical and powerful approach to multiple testing. J R Stat Soc Series B Stat Methodol, 57, 289–300. https://doi.org/10.1111/j.2517-6161.1995.tb02031.x
[17]. Benjamini, Y., Yekutieli, D., 2001, The control of the false discovery rate in multiple testing under dependency. Ann Stat, 29, 1165–1188. DOI: 10.1214/aos/1013699998.
[18]. Shannon, P., Markiel, A., Ozier, O., Baliga, N. S., Wang, J. T., Ramage, D., et al. 2003, Cytoscape: a software environment for integrated models of biomolecular interaction networks. Genome Res, 13, 2498–2504. doi: 10.1101/gr.1239303.
[19]. Szklarczyk, D., Gable, A. L., Lyon, D., Junge, A., Wyder, S., Huerta-Cepas, J., 2019, STRING v11: protein-protein association networks with increased coverage, supporting functional discovery in genome-wide experimental data sets. Nucleic Acids Res, 47, D607-D613. doi: 10.1093/nar/gky1131.
[20]. Chin, C. H., Chen, S. H., Wu, H. H., Ho, C. W., Ko, M. T., Lin, C. Y., 2014, cytoHubba: identifying hub objects and sub-networks from complex interactome. BMC Syst Biol, 8, S11. doi: 10.1186/1752-0509-8-S4-S11.
[21]. Yu, G., Wang, L. G., Han, Y., He, Q. Y., 2021, ClusterProfiler: an R package for comparing biological themes among gene clusters. OMICS, 16, 284–287. doi: 10.1089/omi.2011.0118.
[22]. Ashburner, M., Ball, C. A., Blake, J. A., Botstein, D., Butler, H., Cherry, J. M., et al., 2000, Gene ontology: tool for the unification of biology. The Gene Ontology Consortium. Nat Genet, 25, 25–29. doi: 10.1038/75556.
[23]. Kanehisa, M., Goto, S., 2000, KEGG: kyoto encyclopedia of genes and genomes. Nucleic Acids Res, 28, 27–30. doi: 10.1093/nar/28.1.27.
[24]. Diamanti-Kandarakis, E., Dunaif, A., 2012, Insulin resistance and the polycystic ovary syndrome revisited: an update on mechanisms and implications. Endocr Rev, 33, 981–1030. doi: 10.1210/er.2011-1034.
[25]. Bharali, M. D., Rajendran, R., Goswami, J., Singal, K., Rajendran, V., 2022, Prevalence of Polycystic Ovarian Syndrome in India: A Systematic Review and Meta-Analysis. Cureus, 14, e32351. doi: 10.7759/cureus.32351.
[26]. Zhang, J., Bao, Y., Zhou, X., Zheng, L., 2019, Polycystic ovary syndrome and mitochondrial dysfunction. Reprod Biol Endocrinol, 17, 67. doi: 10.1186/s12958-019-0509-4
[27]. Karakas, S. E., Sun, W. L., 2019, Role of insulin resistance and hyperandrogenemia in the pathogenesis of polycystic ovary syndrome. Fertil Steril, 91, 1063–1071.
[28]. Rosenfield, R. L., 2015, The diagnosis of polycystic ovary syndrome in adolescents. Pediatrics, 136, 1154–1165. doi: 10.1542/peds.2015-1430.
[29]. Mukherjee, A. G., Wanjari, U. R., Kannampuzha, S., Murali, R., Namachivayam, A., Ganesan, R., Dey, A., Babu, A., Renu, K., Vellingiri, B., et al., 2023, The implication of mechanistic approaches and the role of the microbiome in polycystic ovary syndrome (PCOS): A review. Metabolites, 13, 129. doi.org/10.3390/metabo13010129.
[30]. Kanehisa, M., Goto, S., Sato, Y., Furumichi, M., Tanabe, M., 2012, KEGG for integration and interpretation of large-scale molecular data sets. Nucleic Acids Res, 40, D109-14.
[31]. Szklarczyk, D., Gable, A. L., Lyon, D., Junge, A., Wyder, S., Huerta-Cepas, J., et al., 2019, STRING v11: protein-protein association networks with increased coverage, supporting functional discovery in genome-wide experimental datasets. Nucleic Acids Res, 47, D607–D613. doi: 10.1093/nar/gky1131.