Efficient Multilayer Convolutional Models for Abnormal Heartbeat Signal Detection
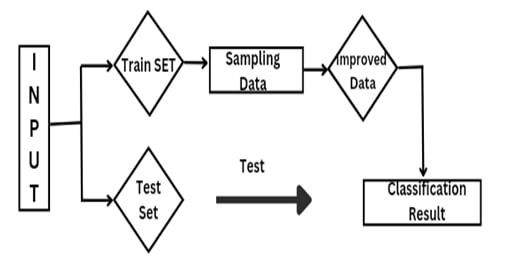
Abstract:
The
escalating ubiquity of heart abnormality extensively, coupled with a
multistorey mortality rate, underscores the crucial need for instantaneous and
efficacious characteristic measures. Recognizing the censorious nature of this
health concern, there's an increasing ultimatum for procedure and machine
techniques that can expeditiously and exactly associate these ailments. The
reason is to plan a mechanized technique category irregular beat sound prompt
to help the surgeon. To the leading of our information, often primary analysis
about that employment a single neural organize show sort of 8 diverse sorts of
pulse sound signal. In an electrocardiogram (ECG), the electrical action of the
heart is recorded and usually spoken to graphically as an arrangement of waves.
The ordinary frequency range for an ECG signal is within the run of 0.05 to 150
Hz. The low-frequency components of the ECG signal (0.05 to 1 Hz) constitute
the pattern or the slow-changing components of the heart's electrical action.
The high-frequency components (1 to 150 Hz) capture the fast changes related to
the depolarization and repolarization of the heart's chambers. The recommended
show is collated with CNN multilayer perceptron (MLP) in diverse execution
assessment lattices. Besides, the results of machine learning (ML) models are
moreover examined. Scheduled show accomplished increases classification acc.
(multiple layers with dropout) acc. 99.35 (single layer with drop) acc. 98.69
(single layer with no drop) acc. 98.18 (multiple layers with no drop) acc.
99.04 dispute collection of data, which is reliably predominant to its
contestant approaches. Representation distributes vital advice to the vascular
specialist identifying heart spout ailment.
References:
[1] Shivnarayan Patidar, Ram Bilas Pachori, 2014, Classification of Cardiac
Sound Signals Using Constrained Tunable-Q Wavelet Transform”, Expert Systems with Applications, Volume 41, Issue 16, 7161-7170, https://doi.org/10.1016/j.eswa.2014.05.052
[2] Manab K., Das corresponding author and Samit Ari, 2014 ,
Patient-specific ECG Beat Classification Technique, National Library of Medicine,
Doi: 10.1049/htl.2014.0072, https://www.ncbi.nlm.nih.gov/pmc/articles/PMC4611171/
[3] lvarado, A. S., Lakshminarayan, C., Principe, J. C, 2012, Time-Based
Compression and Classification of Heartbeats, IEEE
Transactions on Biomedical Engineering
59, 1641–1648, Doi: 10.1109/TBME.2013.2238191, https://ieeexplore.ieee.org/document/6409262?denied=
[4] de Chazal, P., Reilly, R. B, 2006, A Patient-Adapting Heartbeat
Classifier Using ECG Morphology and Heartbeat Interval Features, IEEE Transactions on Biomedical Engineering 53, 2535–2543, Doi: 10.1109/TBME.2006.883802, https://ieeexplore.ieee.org/document/4015601
[5] Christov, I., Jekova, I., Bortolan, G, 2005, Premature Ventricular
Contraction Classification by the kth Nearest-Neighbours Rule, Physiological Measurement 26, 123, Doi: 10.1088/0967-3334/26/1/011, https://iopscience.iop.org/article/10.1088/0967-3334/26/1/011/meta
[6] Hassaan Malik, Umair Bashir, Adnan Ahmad, 2022, Multi-Classification
Neural Network Model for Detection of Abnormal Heartbeat Audio signal, Volume
4, 100048, https://doi.org/10.1016/j.bea.2022.100048
[7] Velagapudi Swapna Sindhu, Kavuri Jaya Lakshmi, Ameya Sanjanita
Tangellamudi, K. Ghousiya Begum, 2023, A Novel Deep Neural Network Heartbeats
Classifier for Heart Health Monitoring, Volume 4, 1-10, https://doi.org/10.1016/j.ijin.2022.11.001
[8] Omair Rashed Abdulwareth Almanifi, Ahmad Fakhri Ab Nasir, Mohd Azraai
Mohd Razman, Rabiu Muazu Musa, Anwar, P. P., AbdulMajeed, 2022, Heartbeat Murmurs
Detection in Phonocardiogram Recordings via
Transfer Learning, Journal 61, 10995–11002, https://doi.org/10.1016/j.aej.2022.04.031
[9] Pratik Kanania, Mamta Padoleb, 2020, ECG Heartbeat Arrhythmia
Classification using Time-Series Augmented Signals and Deep Learning Approach, Procedia Computer Science 171, 524–531, https://doi.org/10.1016/j.procs.2020.04.056
[10] Manish Sharma, Ru-San Tan, U. Rajendra Acharya, 2019, Automated Heartbeat
Classification and Detection of Arrhythmia using Optimal Orthogonal Wavelet
Filters, Informatics in Medicine
Unlocked 16, 100221, https://doi.org/10.1016/j.imu.2019.100221
[11] Aashay Gondalia a, Dhruv Dixit b, Shubham Parashar c, Vijayanand
Raghava d, Animesh Sengupta e, Vergin Raja Sarobin, 2018, IoT-based Healthcare
Monitoring System for War Soldiers using Machine Learning, Procedia Computer Science, Volume 133, 1005-1013, https://doi.org/10.1016/j.procs.2018.07.075
[12] Edward, B., Panganiban, a., Arnold, C., Paglinawan, b., Wen Yaw Chung c.,
Gilbert Lance S., Paa, d., 2021, ECG Diagnostic Support System (EDSS): A Deep
Learning Neural Network Based Classification System for Detecting ECG Abnormal
Rhythms from a Low-Powered Wearable Biosensors, Sensing
and Bio-Sensing Research, Volume 31, 100398, https://doi.org/10.1016/j.sbsr.2021.100398
[13] Jonas Chromik, Lukas Pirl, Jossekin Beilharz, Bert Arnrich, Andreas
Polze, 2021, Certainty in QRS Detection with Artificial Neural Networks, IEEE Conference,
Volume 68, 102628, https://doi.org/10.1016/j.bspc.2021.102628
[14] Zhongwei Jiang, Samjin Choi, 2006, A Cardiac Sound Characteristic
Waveform Method for in-home Heart Disorder Monitoring with Electric
Stethoscopes, Volume 31, Issue 2, 286-298, https://doi.org/10.1016/j.eswa.2005.09.02510
[15] D. Kumar, P. Carvalho, M. Antunes, P. Gil, J. Henriques, L. Eugenio, 2006,
A New Algorithm for Detection of S1 and S2 Heart Sounds”, IEEE Conference,
DOI:10.1109/ICASSP.1660559, https://www.semanticscholar.org/paper/A-New-Algorithm-for-Detection-of-S1-and-S2-Heart-Kumar-Carvalho/120916432fa36d0c2c0d1d1a685a682eae42080d
[16] De Lannoy, G., Francois, D., Delbeke, J., Verleysen, M, 2012, Weighted Conditional
Random Fields for Supervised Interpatient Heartbeat Classification, IEEE Transactions on Biomedical Engineering, Doi: 10.1109/TBME.2011.2171037, https://pubmed.ncbi.nlm.nih.gov/21990327/
[17] Guler, I., Ubeylı, E. D, 2005, ECG Beat Classifier Designed by Combined
Neural Network Model, Pattern Recognition 38(2):199-208, Doi:10.1016/S0031-3203(04)00276-6,
https://www.researchgate.net/publication/271584317_ECG_beat_classifier_designed_by_combined_neural_network_model
[18] Das, M. K., Ari, S., 2014, Patient-Specific ECG Beat Classification
Technique, Healthcare Technology Letters, PMID: 26609386 PMCID: PMC4611171 DOI:
10.1049/htl.2014.0072 https://pubmed.ncbi.nlm.nih.gov/26609386/
[19] Hu, Y. H., Palreddy, S., Tompkins, W. J, 1997, A Patient-Adaptable ECG Beat
Classifier Using A Mixture of Experts Approach, IEEE
Transactions on Biomedical Engineering 44,
891–900, DOI 10.1109/10.623058, https://ieeexplore.ieee.org/document/623058
[20] Huang, H., Liu, J., Zhu, Q., Wang, R., Hu, G, 2014, Detection of
Inter-Patient Left and Right Bundle Branch Block Heartbeats in ECG using Ensemble
Classifiers, Biomedical Engineering
Online 13, 72, https://doi.org/10.1186/1475-925X-13-72
[21] Huang, H., Liu, J., Zhu, Q., Wang, R., Hu, G., 2014, A New Hierarchical
Method for Inter-Patient Heartbeat Classification using Random Projections and
rr intervals, Biomedical Engineering Online
13, 90, https://doi.org/10.1186/1475-925X-13-90
[22] Ince, T., Kiranyaz, S., Gabbouj, M., 2009, A Generic and Robust System
for Automated Patient-Specific Classification of ECG signals, IEEE Transactions on Biomedical Engineering 56, 1415–1426, DOI: 10.1109/TBME.2009.2013934, https://ieeexplore.ieee.org/document/4776456
[23] Jacobs, R. A., 1995, Methods for Combining Experts’ Probability
Assessments”, Neural Computation 7, 867–888, https://direct.mit.edu/neco/article-abstract/7/5/867/5891/Methods-For-Combining-Experts-Probability?redirectedFrom=fulltext
[24] Liao, T. W., 2005, Clustering of Time Series Data—A Survey, Pattern Recognition
38, 1857–1874, https://doi.org/10.1016/j.patcog.2005.01.025
[25] Llamedo, M., Mart ́ınez, J. P., 2011, Heartbeat Classification Using
Feature Selection Driven by Database Generalization Criteria, IEEE Transactions on Biomedical Engineering 58, 616–625.DOI: 10.1109/TBME.2010.2068048, https://ieeexplore.ieee.org/document/5551181
[26] Salem, A. B. M., Revett, K., El-Dahshan, E., 2009. Machine Learning in
Electrocardiogram Diagnosis. International Multiconference on Computer Science and
Information Technology, https://www.proceedings2009.imcsit.org/pliks/10.pdf