Statistics and Biomedical Research
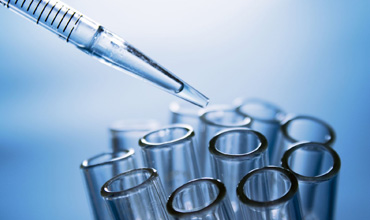
Abstract:
Biostatistics
is the application of statistical techniques to biological data obtained
prospectively and/or retrospectively. Statistics plays critical analytical role
in biomedical research. It is the bases for building clear inference from the
data collected in a biomedical evaluation and without which it would be
impossible to declare an outcome from any clinical trial. This critical role of
biostatistics in biomedical research was noted by Cadarso-Suárez, and González-Manteiga, (2007), who stated that
“the discipline of biostatistics is nowadays a fundamental scientific component
of biomedical, public health and health services research” and pointed out
traditional and emerging areas of application as “clinical trials research,
observational studies, physiology, imaging, and genomics”.
At
the same time, misuse of biostatistics has resulted in several misleading
outcomes and several workers have progressively noted the many statistical
errors and shortcomings found in a large number of biomedical publications
(Porter, 1999; Cooper, et al., 2002; García-Berthou
and Alcaraz 2004; Strasak, et
al., 2007; Ercan, et al., 2007; Thiese, et al., 2015). Ercan, et al., (2007) specifically notes
that this observations cuts across “every
stage of a medical research related to data analysis; design of the experiment,
data collection and pre-processing, analysis method and implementation, and
interpretation”. Similarly, Thiese, et al., (2015), points to data
abuses such as “incorrect application of statistical tests, lack of transparency
and disclosure about decisions that are made, incomplete or incorrect
multivariate model building, or exclusion of outliers”.
The
role of statistics in medical research starts at the planning stage of a
clinical trial or laboratory experiment to establish the design and size of an
experiment that will ensure a good prospect of detecting effects of clinical or
scientific interest. Statistics is again used during the analysis of data
(sample data) to make inferences valid in a wider population. Specifically,
statistics has two roles in laboratory experiments and clinical trials.
References:
1. Abdolell
M., LeBlanc M., Stephens D., et al. Binary partitioning for continuous
longitudinal data: categorizing a prognostic variable. Statistics in Medicine
2002;21:3395–3409. [PubMed: 12407680]
2. Altman
D. G., Lausen B, Sauerbrei W, et al. Dangers of using “optimal” cutpoints in
the evaluation of prognostic factors. Journal of the National Cancer Institute
1994;86:829–835. [PubMed: 8182763]
3.
Bossuyt, P.M., Reitsma, J.B., Bruns,
D.E., Gatsonis C.A., Glasziou, P.P., Irwig, L.M., et al. The STARD statement
for reporting studies of diagnostic accuracy: explanation and elaboration. Ann
Intern Med 2003;138:W1-12. http://dx.doi.org/10.7326/0003-4819-138-1-200301070-00010.
4.
Cadarso-Suárez, C. and González-Manteiga W. (2007).
Statistics In Biomedical Research; ARBOR
Ciencia, Pensamiento y Cultura CLXXXIII 725 353-361 ISSN: 0210-1963
5. Chansky,
K., Sculier, J.P., Crowley, J.J., et al. The International Association for the
Study of Lung Cancer Staging Project: prognostic factors and pathologic TNM
stage in surgically managed non-small cell lung cancer. J Thorac Oncol
2009;4(7):792–801. [PubMed: 19458556]
6. Cooper,
R.J., Schriger D.L., Close R.J.H. Graphical literacy: the quality of graphs in
a large-circulation journal. Ann Emerg Med. 2002;40:317–22.
7.
Ercan I., Yazıcı B., Yang, Y., Özkaya G., Cangur S., Ediz, B., Kan, I.,
(2007); Misusage of Statistics in Medical Research. Eur J
Gen Med; 4(3):128-134
8. García-Berthou
E., Alcaraz C., Incongruence between test statistics and P values in medical
papers. BMC Med Res Method. 2004;4:13–7.
9. Harrell,
F.E., Jr. Regression modelling strategies with applications to linear models,
logistic regression, and survival analysis. Springer-Verlag; New York: 2001.
10. Mandrekar, J. N. and
Mandrekar, S. J., (2009). Biostatistics: A toolkit for exploration,
validation and interpretation of clinical data. J Thorac Oncol.;
4(12): 1447–1449. doi:10.1097/JTO.0b013e3181c0a329.
11. Mazumdar,
M., Glassman, J. R., Categorizing a prognostic variable: review of methods,
code for easy implementation and applications to decision-making about cancer
treatments. Statistics in Medicine 2000;19:113–132. [PubMed: 10623917]
12. Moher,. D.,
Schulz, K.F., Altman DG. The CONSORT statement: revised recommendations for
improving the quality of reports of parallel group randomized trials. BMC Med
Res Methodol 2001;1:2. http://dx.doi.org/10.1186/1471-2288-1-2.
13. Porter,
A. M., Misuse of correlation and regression in three medical journals. J Roy
Soc Med. 1999;92:123–8.
14. Sprent,
P., Statistics in medical research. Swiss Med Wkly 2003;133(3940):522–9.
[PubMed: 14655052]
15. Strasak, A. M., Zaman, Q., Pfeiffer, K. P., Göbel,
G., Ulmer, H., (2 0 0 7). Statistical errors in
medical research –a review of common pitfalls SWISS MED WKLY; 137:44–49 www.smw.ch
16. Thiese, M. S., Arnold, Z. C., Walker,
S. D. (2015). The misuse and abuse of statistics in biomedical research: Biochemia
Medica 2015;25(1):5–11 http://dx.doi.org/10.11613/BM.2015.001
17. Von Elm E.,
Altman D.G., Egger M., Pocock, S.J., Gotzsche, P.C., Vandenbroucke, J.P., The
Strengthening the Reporting of Observational Studies in Epidemiology (STROBE)
statement: guidelines for reporting observational studies. Prev Med
2007;45:247-51. http://dx.doi.org/10.1016/j.ypmed.2007.08.012.